How to deploy natural language to SQL on your own data – in just one hour with Kinetica SQL-GPT
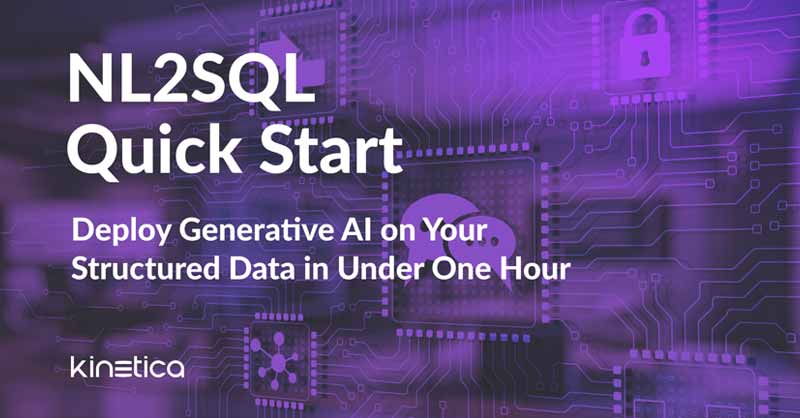
You’ve seen how Kinetica enables generative AI to create working SQL queries from natural-language questions, using data set up for the demonstration by Kinetica engineers. What about your data? How can you make Kinetica respond to real SQL queries about data that belongs to you, that you work with today, using conversational, natural-language questions, right now? You’re about to see how Kinetica SQL-GPT enables you to have a conversation with your own data. Not ours, but yours. With the built-in SQL-GPT demos, the data is already imported, and the contexts that help make that data more associative with natural language, already entered. When your goal is to make your own data as responsive as the data in our SQL-GPT demos, there are steps you need to take first. This page shows you how to do the following: STEP 1: Import your Data into Kinetica Kinetica recognizes data files stored in the following formats: delimited text files (CSV, TSV), Apache Parquet, shapefiles, JSON, and GeoJSON [Details]. For Kinetica to […]
The mission to make data conversational
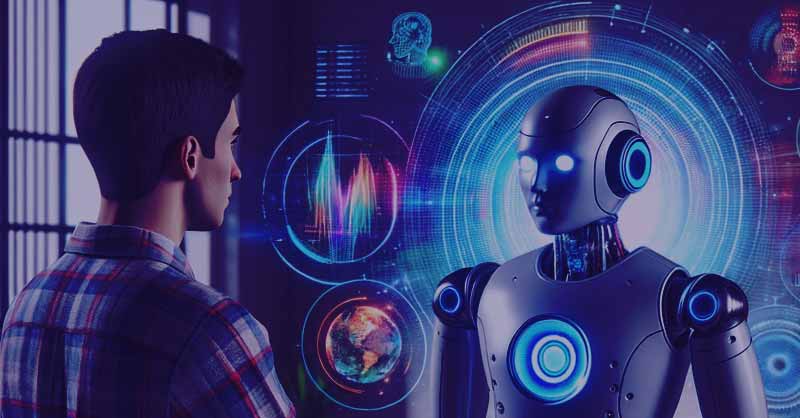
I think one of the most important challenges for organizations today is to use the data they already have more effectively, in order to better understand their current situation, risks, and opportunities. Modern organizations accumulate vast amounts of data, but they often fail to take full advantage of it because they struggle finding the right skilled resources to analyze it that would unlock critical insights. Kinetica provides a single platform that can perform complex and fast analysis on large amounts of data with a wide variety of analysis tools. This, I believe, makes Kinetica well-positioned for data analytics. However, many analysis tools are only available to users who possess the requisite programming skills. Among these, SQL is one of the most powerful and yet it can be a bottleneck for executives and analysts who find themselves relying on their technical teams to write the queries and process the reports. Given these challenges Nima Neghaban and I saw an opportunity for AI models to generate SQL based on natural […]
Towards long-term memory recall with Kinetica, an LLM, and contexts
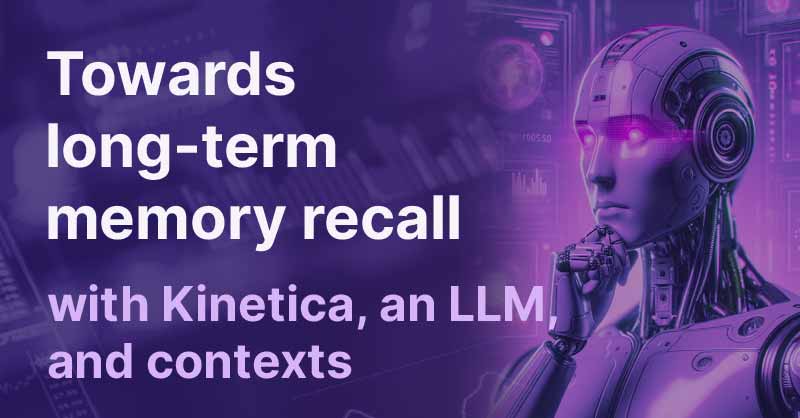
Prior to the emergence of machine learning, and particularly “deep learning,” I was an ML skeptic. Judging from what I saw from the state of the art at the time, I’d say there was no way to program a CPU or a GPU — each of which, after all, is just a sophisticated instance of a Turing machine — to make it exhibit behaviors that could pass for human intelligence. It seemed like a sensible enough stance to take, given that I spent the bulk of a typical work week translating ambiguous requirements from customers into unambiguous instructions a computer could execute. Algorithmic neural networks had been around since the 1950s, yet most AI algorithms had been designed to follow a fixed set of steps with no concept of training. Algorithms are sets of recursive steps that programs should follow to attain a discrete result. While machine learning does involve algorithms at a deep level, what the computer appears to learn from ML typically does not follow any […]
Get Started with the JDBC Connector
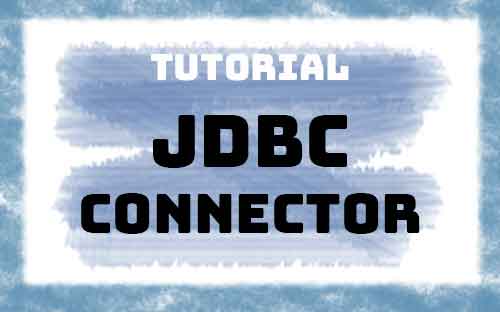
This tutorial will walk you through how to get started accessing Kinetica with the JDBC Connector. In addition to explaining the core JDBC API best practices it will cover important functionality that is specific to Kinetica. We will start by importing the tutorial project into eclipse and configuring the JDBC connection to use your local Kinetica instance. Next the tutorial will walk you through JUnit test cases that provide working examples that include: Creating a table Inserting and updating rows Querying for data Handling Kinetica specific datatypes Finally, after you complete the tutorial the examples may be useful for troubleshooting issues encountered in the field. Prerequisites This tutorial assumes that you… Have a Java IDE. We will use Eclipse in the examples but others should work. Have access to a Kinetica 7.1 environment where you have permissions for create/insert/update of tables. If you don’t have this then you can get Kinetica Developer Edition which is free to try. Have familiarity with Java and SQL. When in doubt about […]
Tutorial – Interactive 3D Visualizations of Massive Datasets
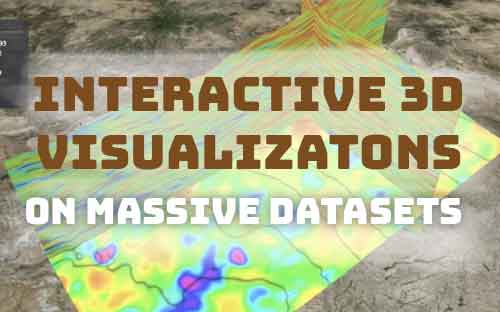
Introduction For companies engaged in oil and gas exploration, getting fast access to high resolution data is an important enabler for finding the right locations to drill a well before their competitors. We worked to pioneer a solution for interactive 3D visualizations of oil basins using datasets containing over 100 billion data points – as described in Oil & Gas Engineering article and the video below The customer solution made use of the vectorized processing capabilities of GPUs for fast visualizations at scale. This tutorial will run you through how you can use Kinetica Developer Edition to create similar 3D models with a demo dataset and just a laptop. Analyzing the Basin Data When a decision is made to drill a well, the costs increase rapidly as competitors buy up properties inside the basin. Each well costs millions of dollars to drill and the revenue produced by the well needs to exceed the production costs. Inaccurate or late decisions can result in substantial losses. Finding the ideal well […]
Kinetica Sparse Data Tutorial
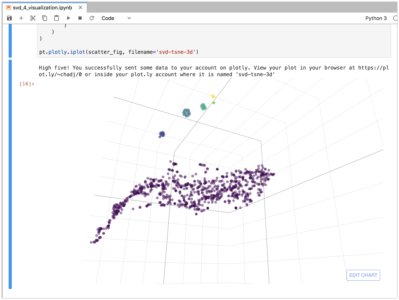
Introduction This tutorial describes the application of Singular Value Decomposition or SVD to the analysis of sparse data for the purposes of producing recommendations, clustering, and visualization on the Kinetica platform. Sparse data is common in industry and especially in retail. It often results when a large set of customers make a small number of choices from a large set of options. Some examples include product purchases, movie rentals, social media likes, and election votes. The SVD approach to analyzing sparse data has a notable history of success. In 2006 Netflix hosted a million dollar competition for the best movie recommendation algorithm and most of the leading entries used SVD. It also inspired the techniques used by Cambridge Analytica when they assisted Trump in the 2016 presidential election. We will leverage Python and Jupyter notebooks with some ML libraries including Scikit Learn and PyTorch. Data will be persisted in Kinetica tables and we will use Kinetica for calculating dot products necessary for inferencing. We start by downloading a […]
Kinetica with JupyterLab Tutorial
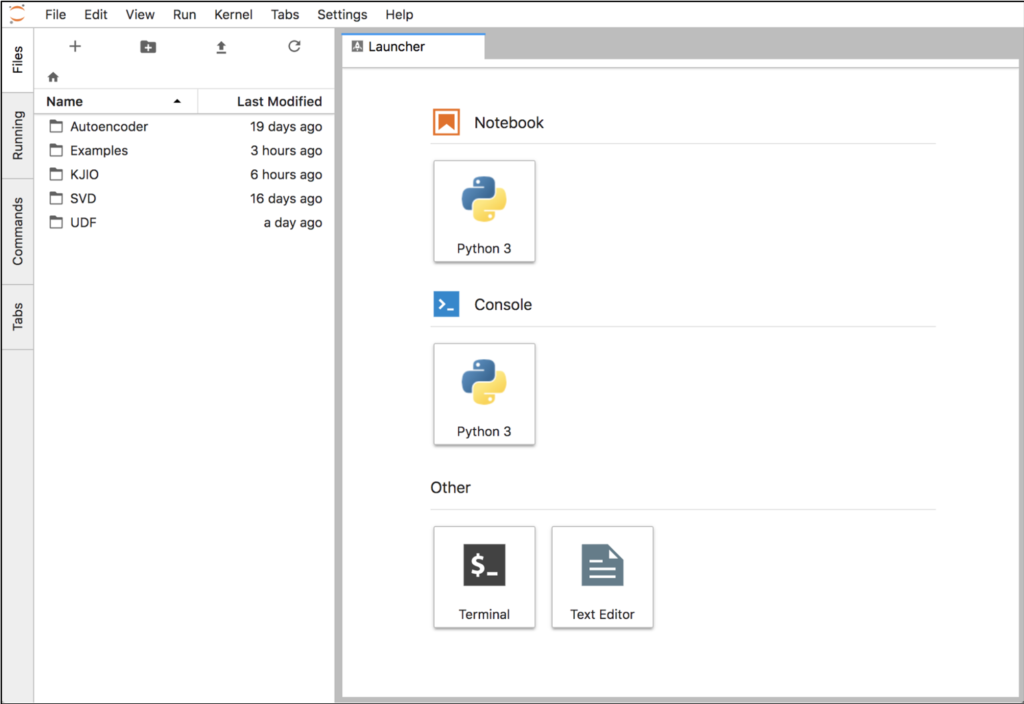
Introduction JupyterLab is an integrated environment that can streamline the development of Python code and Machine Learning (ML) models in Kinetica. With it you can edit Jupyter notebooks that integrate code execution, debugging, documentation, and visualization in a single document that can be consumed by multiple audiences. The development process is streamlined because sections of code (or cells) can be run iteratively while updating results and graphs. It can be accessed from a web browser and supports a Python console with tab completions, tooltips, and visual output. One of the difficulties of using Jupyter notebooks with Kinetica had been that an environment needs to be installed with all the necessary dependencies. In this tutorial we will simplify this process with a Docker image that integrates the components so they can run locally on any Intel-based machine. The image integrates the following major components: CentOS 7 Kinetica 6.2 JupyterLab Python 3.6 The Python environment has the necessary modules for: Interaction with Kinetica using ODBC or the native API Creating […]